Data Analysis For Finance
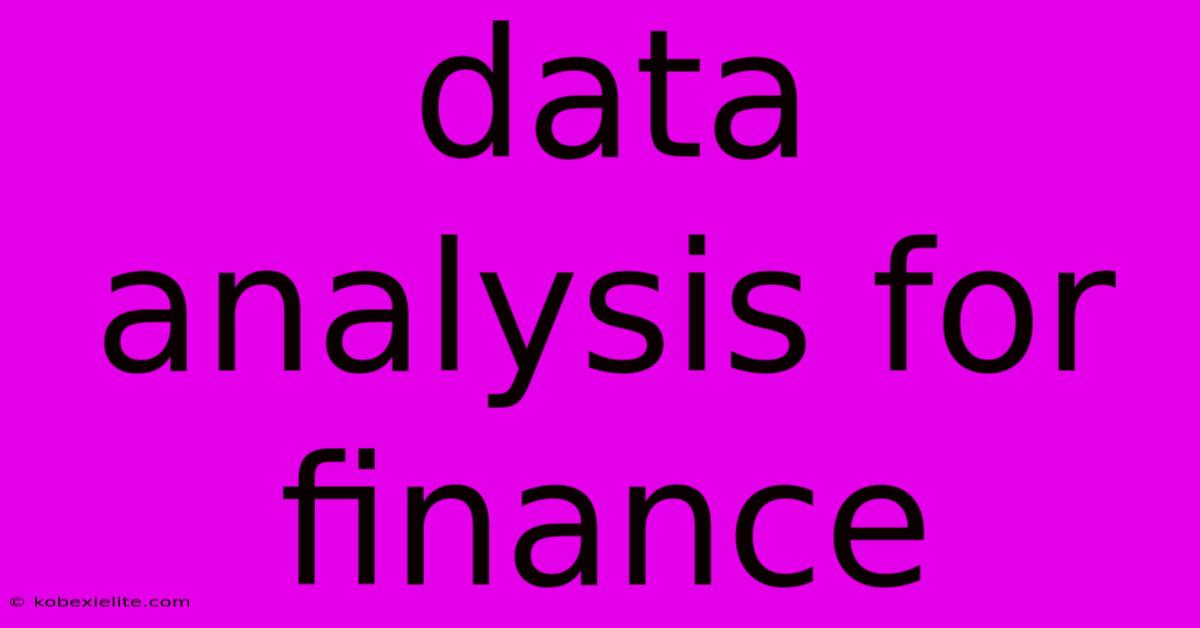
Discover more detailed and exciting information on our website. Click the link below to start your adventure: Visit Best Website mr.cleine.com. Don't miss out!
Table of Contents
Data Analysis for Finance: Unlocking Financial Insights
The financial world is awash in data. From stock prices and market trends to customer transactions and risk assessments, information is the lifeblood of successful financial institutions and savvy investors. This is where data analysis for finance comes in, transforming raw data into actionable insights that drive informed decision-making. This comprehensive guide explores the crucial role of data analysis in the finance industry, examining its applications, techniques, and the skills needed to excel in this dynamic field.
Why is Data Analysis Crucial in Finance?
In today's competitive financial landscape, relying solely on intuition and gut feelings is a recipe for disaster. Data analysis for finance offers a powerful antidote, providing a rigorous, evidence-based approach to:
- Identifying profitable investment opportunities: By analyzing historical market data, economic indicators, and company performance metrics, analysts can pinpoint undervalued assets and potential growth areas.
- Managing risk effectively: Sophisticated data analysis techniques help assess and mitigate various financial risks, including credit risk, market risk, and operational risk. This involves identifying potential threats and developing strategies to minimize their impact.
- Improving operational efficiency: Analyzing internal data, such as transaction data and customer behavior, can optimize processes, reduce costs, and improve overall operational efficiency within financial institutions.
- Detecting fraudulent activities: Data analysis plays a vital role in identifying anomalies and patterns indicative of fraudulent transactions, helping protect financial institutions and their clients from financial losses.
- Enhancing customer experience: By analyzing customer data, financial institutions can personalize services, improve customer support, and build stronger customer relationships.
Key Techniques in Financial Data Analysis
Several key techniques are frequently employed in financial data analysis:
1. Descriptive Statistics:
This foundational aspect involves summarizing and visualizing data to understand its basic characteristics. Techniques include calculating mean, median, mode, variance, and standard deviation. Descriptive statistics are essential for providing a preliminary understanding of the data before moving on to more advanced analyses.
2. Regression Analysis:
Regression analysis is a powerful tool used to model the relationship between different variables. In finance, it's widely applied to predict future returns, assess the impact of economic factors on stock prices, or evaluate the effectiveness of investment strategies. Linear regression, multiple regression, and logistic regression are common examples.
3. Time Series Analysis:
Financial data often has a time component. Time series analysis focuses on understanding patterns and trends in data over time. Techniques like moving averages, exponential smoothing, and ARIMA models are used to forecast future values, such as stock prices or interest rates.
4. Machine Learning:
Machine learning algorithms are increasingly being employed in finance for tasks such as fraud detection, algorithmic trading, and credit scoring. These algorithms can identify complex patterns and relationships in large datasets that may be missed by traditional statistical methods. Examples include decision trees, support vector machines, and neural networks.
5. Monte Carlo Simulation:
This technique involves running numerous simulations to model the probability of different outcomes. In finance, Monte Carlo simulations are used to assess the risk associated with investments and to evaluate the potential performance of portfolios under various market conditions.
Essential Skills for Financial Data Analysts
To succeed in this field, professionals need a blend of technical and soft skills:
- Proficiency in programming languages: Python and R are highly sought-after languages for financial data analysis due to their extensive libraries for statistical computing and data visualization.
- Strong statistical knowledge: A solid understanding of statistical concepts and techniques is crucial for interpreting data accurately and drawing meaningful conclusions.
- Expertise in database management: The ability to extract, clean, and manage large financial datasets is essential for effective data analysis.
- Data visualization skills: Effectively communicating findings through clear and concise visualizations is key to influencing decision-making.
- Business acumen: Understanding the financial industry and the business context of data analysis is vital for providing relevant and actionable insights.
- Communication skills: The ability to explain complex analytical findings to both technical and non-technical audiences is a highly valued skill.
The Future of Data Analysis in Finance
The future of data analysis for finance is bright, with continued advancements in technology and the increasing availability of data. The integration of big data, artificial intelligence, and cloud computing is transforming the field, enabling more sophisticated analyses and creating new opportunities for innovation. As the financial world becomes increasingly data-driven, professionals skilled in data analysis for finance will be in high demand. The ability to extract meaningful insights from complex data will be a critical differentiator for success in this ever-evolving landscape.
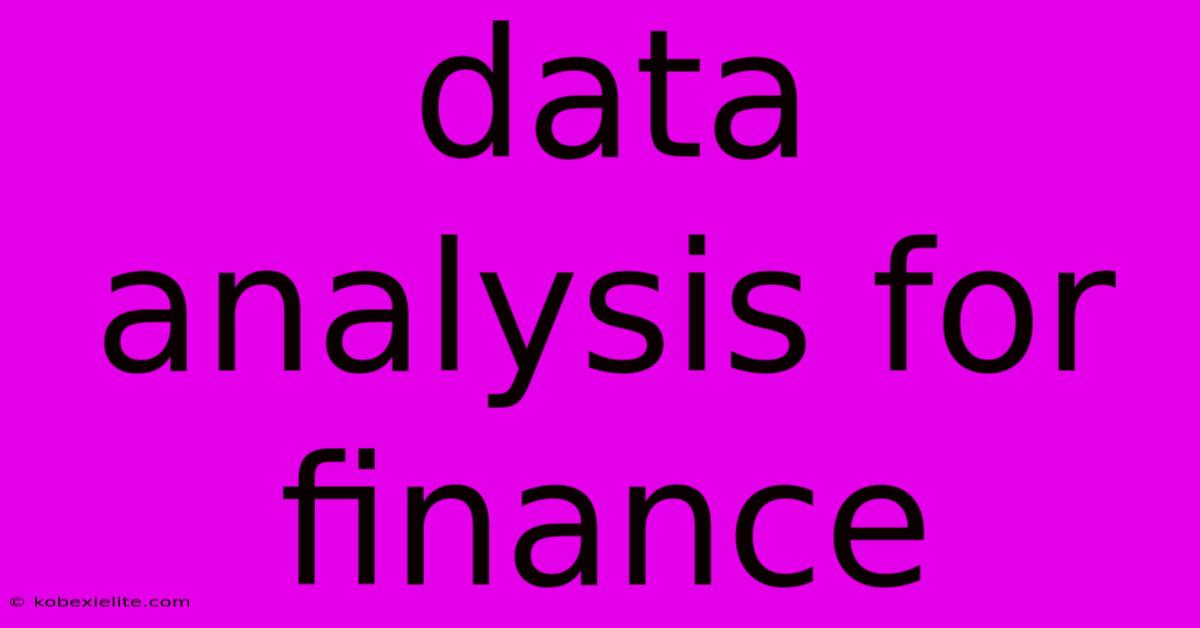
Thank you for visiting our website wich cover about Data Analysis For Finance. We hope the information provided has been useful to you. Feel free to contact us if you have any questions or need further assistance. See you next time and dont miss to bookmark.
Featured Posts
-
Ceo Finance Academy Reviews
Dec 15, 2024
-
Performance Management Finance
Dec 15, 2024
-
Sixers Rookie Mc Cain Indefinite Meniscus Injury
Dec 15, 2024
-
Ter Finance
Dec 15, 2024
-
Master Finance Columbia
Dec 15, 2024