Data Analysis In Finance
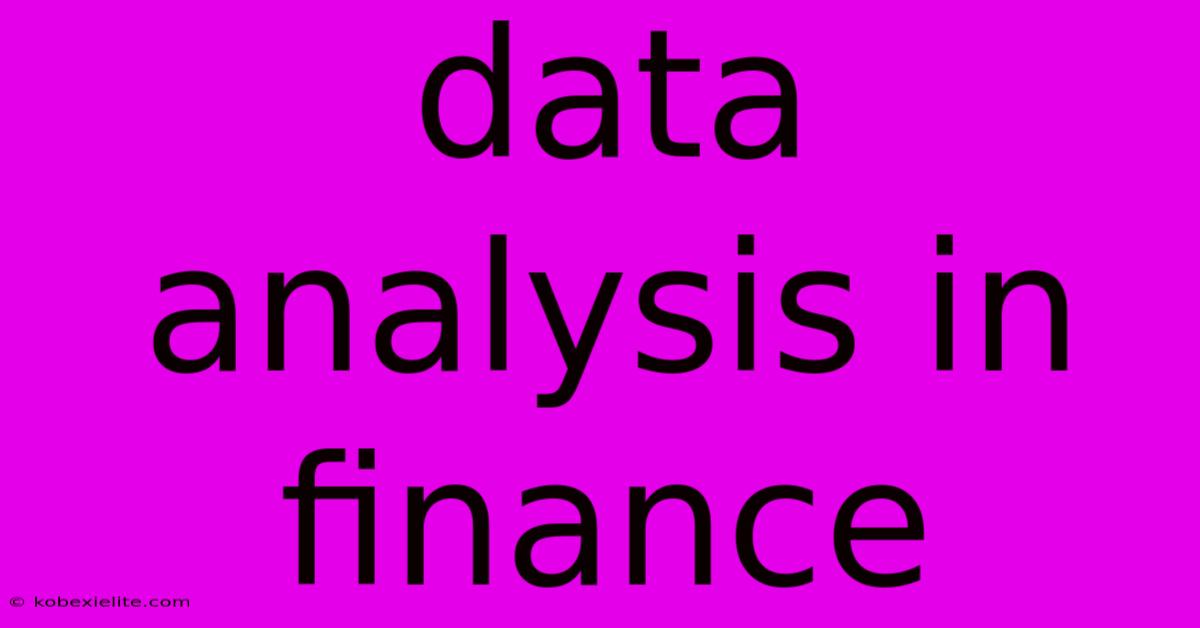
Discover more detailed and exciting information on our website. Click the link below to start your adventure: Visit Best Website mr.cleine.com. Don't miss out!
Table of Contents
Data Analysis in Finance: Unlocking Financial Insights
Data analysis has revolutionized the financial industry, transforming how institutions make decisions, manage risk, and identify opportunities. From algorithmic trading to fraud detection, the ability to extract meaningful insights from vast datasets is no longer a luxury but a necessity. This article delves into the crucial role of data analysis in finance, exploring its applications, techniques, and future trends.
The Expanding Landscape of Financial Data Analysis
The financial world generates an unprecedented volume of data daily. This includes transactional data, market data, economic indicators, social media sentiment, and more. Effective data analysis techniques are essential to sift through this information and uncover actionable intelligence.
Key Applications of Data Analysis in Finance:
- Algorithmic Trading: High-frequency trading (HFT) algorithms rely heavily on data analysis to identify and execute trades at optimal prices. These algorithms analyze vast amounts of market data in real-time to predict price movements and capitalize on fleeting opportunities.
- Risk Management: Sophisticated models, often employing machine learning techniques, assess and manage various financial risks, including credit risk, market risk, and operational risk. Data analysis helps to identify potential vulnerabilities and develop mitigation strategies.
- Fraud Detection: Financial institutions utilize data analysis to detect fraudulent transactions and activities. Anomaly detection algorithms identify unusual patterns that may indicate fraudulent behavior.
- Portfolio Management: Data analysis plays a crucial role in optimizing investment portfolios. Analyzing historical data, market trends, and economic indicators allows for better asset allocation and risk-adjusted returns.
- Customer Relationship Management (CRM): Understanding customer behavior is vital for financial institutions. Data analysis helps to segment customers, personalize services, and improve customer retention.
- Regulatory Compliance: Financial institutions face stringent regulatory requirements. Data analysis helps to ensure compliance by monitoring transactions, identifying suspicious activities, and generating necessary reports.
Essential Data Analysis Techniques in Finance
Financial data analysis employs a range of statistical and machine learning techniques, including:
- Descriptive Statistics: Summarizing and visualizing data to understand key trends and patterns.
- Regression Analysis: Modeling the relationship between different variables to predict future outcomes.
- Time Series Analysis: Analyzing data collected over time to identify trends and seasonality.
- Classification: Categorizing data into different groups based on specific characteristics (e.g., classifying loan applicants as high-risk or low-risk).
- Clustering: Grouping similar data points together to identify patterns and segments (e.g., grouping customers based on their investment behavior).
- Machine Learning: Using algorithms to learn from data and make predictions or decisions without explicit programming (e.g., using neural networks for fraud detection).
The Future of Data Analysis in Finance
The future of data analysis in finance is bright, driven by several key trends:
- Big Data and Cloud Computing: The ability to process and analyze massive datasets is becoming increasingly crucial. Cloud computing provides the infrastructure needed to handle this volume of data.
- Artificial Intelligence (AI) and Machine Learning: AI and machine learning are transforming the financial industry by automating tasks, improving decision-making, and uncovering new insights.
- Alternative Data Sources: Financial institutions are increasingly using alternative data sources, such as social media sentiment and satellite imagery, to enhance their analysis and gain a competitive edge.
- Explainable AI (XAI): As AI models become more complex, the need for explainable AI is growing. XAI aims to make the decision-making process of AI models more transparent and understandable.
Conclusion:
Data analysis is fundamental to the success of financial institutions in today's competitive landscape. By leveraging advanced techniques and embracing new technologies, financial professionals can unlock valuable insights, manage risk effectively, and drive growth. The ongoing evolution of data analysis will continue to shape the future of finance, creating new opportunities and challenges for the industry.
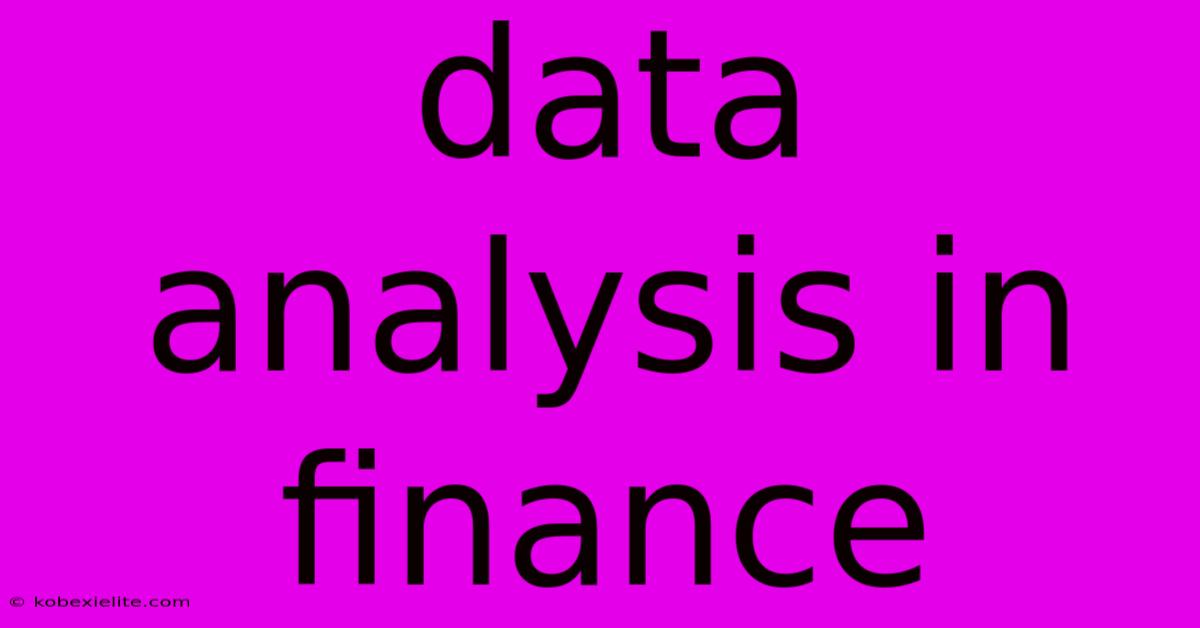
Thank you for visiting our website wich cover about Data Analysis In Finance. We hope the information provided has been useful to you. Feel free to contact us if you have any questions or need further assistance. See you next time and dont miss to bookmark.
Featured Posts
-
Worlds Best 37 Fast Food Chains
Dec 15, 2024
-
Real Madrid Lineup Ancelottis Key Choices
Dec 15, 2024
-
Buckley Upsets Covington At Ufc Tampa
Dec 15, 2024
-
Emirates Cup Semifinal Matches
Dec 15, 2024
-
Gabba Day Two Sunny Start
Dec 15, 2024