Forecasting Techniques In Finance
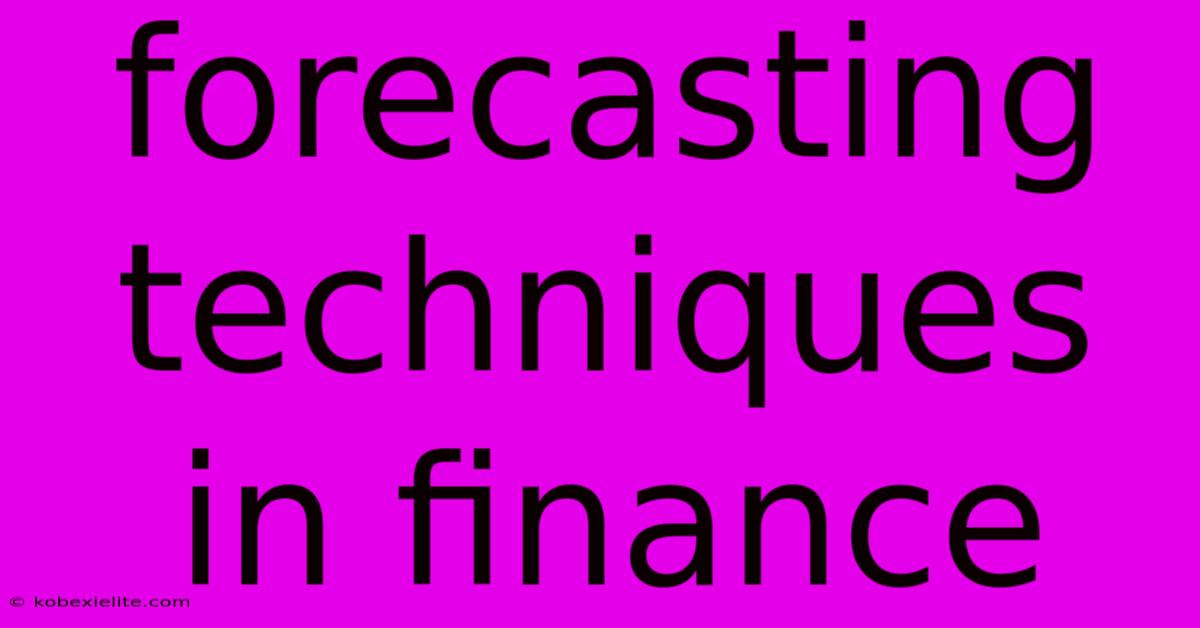
Discover more detailed and exciting information on our website. Click the link below to start your adventure: Visit Best Website mr.cleine.com. Don't miss out!
Table of Contents
Forecasting Techniques in Finance: Predicting Market Trends and Investment Opportunities
Forecasting in finance is the art and science of predicting future financial variables. Accurate forecasting is crucial for informed decision-making across various financial domains, from investing and risk management to budgeting and financial planning. This article explores several key forecasting techniques used by financial professionals.
Understanding the Importance of Financial Forecasting
Financial forecasting isn't about predicting the future with absolute certainty; it's about using data-driven methods to improve the probability of making sound financial decisions. Accurate forecasts can lead to:
- Improved Investment Strategies: Identifying potential market trends and selecting investments poised for growth.
- Effective Risk Management: Assessing and mitigating potential risks associated with investments and financial operations.
- Enhanced Budgeting and Planning: Creating realistic budgets and financial plans based on anticipated revenues and expenses.
- Better Resource Allocation: Optimizing the use of financial resources based on projected needs.
Key Forecasting Techniques in Finance
Several techniques are employed for financial forecasting, each with its own strengths and limitations. Let's explore some of the most commonly used:
1. Time Series Analysis
This quantitative method analyzes historical data to identify patterns and trends that can be extrapolated into the future. Popular time series models include:
- Moving Averages: Calculate the average of a specific number of past data points to smooth out short-term fluctuations and identify underlying trends.
- Exponential Smoothing: Assigns exponentially decreasing weights to older data, giving more importance to recent observations. This is particularly useful for data with trends and seasonality.
- Autoregressive Integrated Moving Average (ARIMA): A sophisticated model that captures complex patterns in time series data, including trends, seasonality, and autocorrelation. Requires significant statistical expertise to implement effectively.
2. Regression Analysis
This statistical technique examines the relationship between a dependent variable (e.g., stock price) and one or more independent variables (e.g., interest rates, inflation). It allows for forecasting the dependent variable based on projected values of the independent variables. Different types of regression analysis include:
- Linear Regression: Assumes a linear relationship between variables.
- Multiple Regression: Uses multiple independent variables to forecast the dependent variable, offering a more comprehensive analysis.
- Nonlinear Regression: Accounts for non-linear relationships between variables.
3. Qualitative Forecasting Methods
These methods rely on expert judgment and subjective assessments rather than solely on quantitative data. They are particularly useful when historical data is limited or unreliable. Examples include:
- Delphi Method: Involves gathering opinions from a panel of experts through multiple rounds of questionnaires, aiming to reach a consensus forecast.
- Scenario Planning: Develops multiple plausible scenarios based on different assumptions about future events. This allows for assessing the potential impact of various uncertainties.
4. Machine Learning Techniques
Recent advancements in machine learning have revolutionized financial forecasting. Algorithms like:
- Neural Networks: Can capture complex, non-linear relationships in data and are particularly effective for high-dimensional datasets.
- Support Vector Machines (SVMs): Powerful tools for classification and regression tasks, useful for predicting market movements and identifying investment opportunities.
Choosing the Right Forecasting Technique
The selection of the appropriate forecasting technique depends on several factors, including:
- Data Availability: The amount and quality of historical data available greatly influences the choice of technique.
- Forecasting Horizon: The time period for which the forecast is needed influences the model selection. Short-term forecasts often use simpler methods, while long-term forecasts often benefit from more complex models.
- Data Characteristics: The presence of trends, seasonality, and other patterns in the data should be considered.
- Computational Resources: Some techniques, like machine learning models, require significant computational power.
Conclusion
Effective financial forecasting is a critical element of successful financial management. By understanding the various techniques available and selecting the most appropriate method based on specific needs, financial professionals can significantly improve their decision-making process and enhance their chances of achieving financial objectives. However, itโs crucial to remember that no forecasting technique guarantees perfect accuracy. Continuous monitoring, adaptation, and a healthy dose of skepticism are essential for navigating the complexities of the financial world.
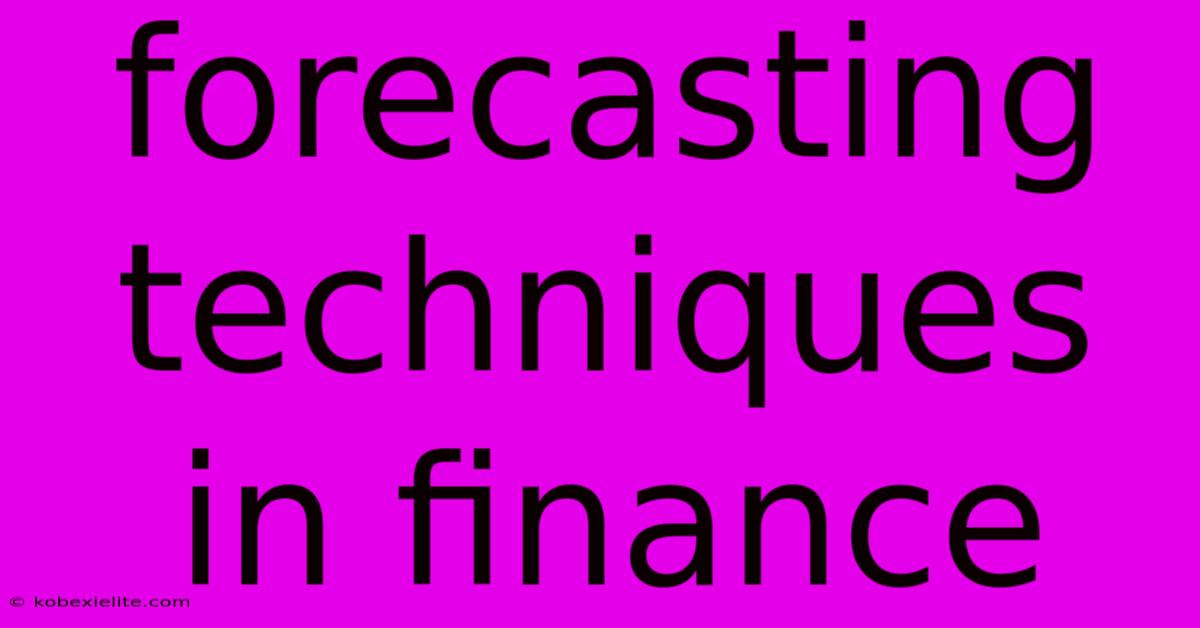
Thank you for visiting our website wich cover about Forecasting Techniques In Finance. We hope the information provided has been useful to you. Feel free to contact us if you have any questions or need further assistance. See you next time and dont miss to bookmark.
Featured Posts
-
Regina Trivia Buffs Win Jeopardy
Dec 15, 2024
-
Covington Finance Near Me
Dec 15, 2024
-
Lecce Vs Monza Prediction And Team News
Dec 15, 2024
-
Awful Start Season Opener Play Stopped
Dec 15, 2024
-
Rolex With Finance
Dec 15, 2024