Machine Learning In Finance From Theory To Practice Pdf
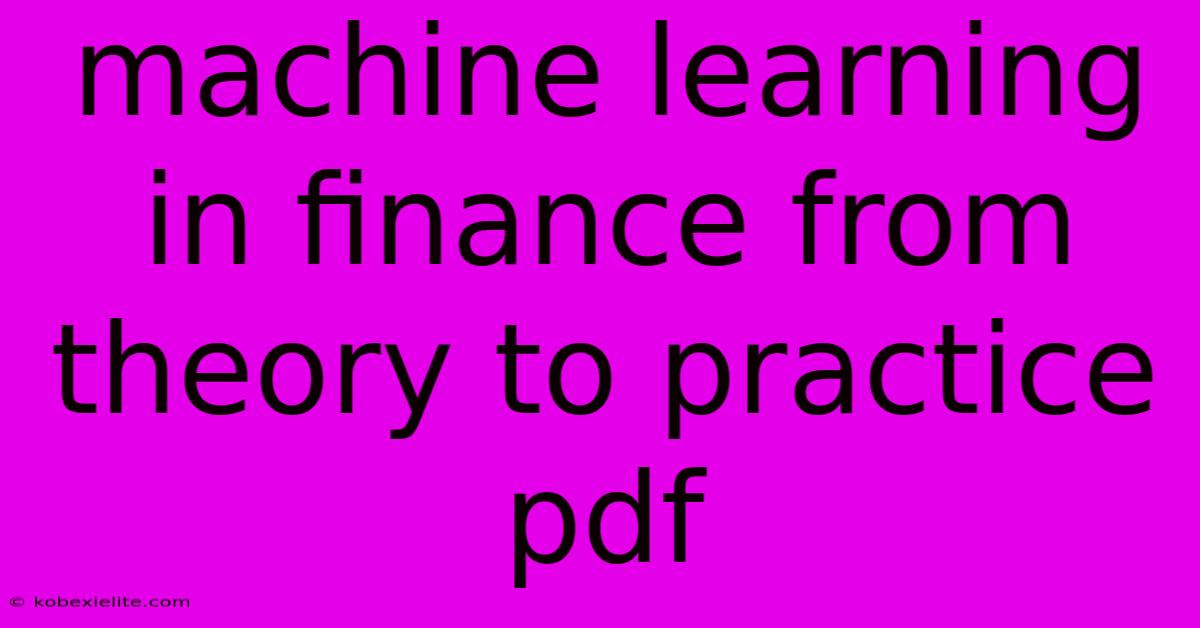
Discover more detailed and exciting information on our website. Click the link below to start your adventure: Visit Best Website mr.cleine.com. Don't miss out!
Table of Contents
Machine Learning in Finance: From Theory to Practice
The financial industry is undergoing a dramatic transformation, fueled by the rapid advancements in machine learning (ML). This powerful technology is no longer a futuristic concept; it's actively shaping investment strategies, risk management, and fraud detection. This article explores the theoretical underpinnings of ML in finance and then delves into practical applications, offering a comprehensive overview of this rapidly evolving field. While a PDF download isn't directly provided here, the information presented will offer a solid foundation equivalent to a substantial portion of such a document.
Theoretical Foundations of Machine Learning in Finance
At its core, ML leverages algorithms to analyze vast datasets, identify patterns, and make predictions without explicit programming. Several key ML techniques are particularly relevant in finance:
1. Supervised Learning:
This approach uses labeled datasets โ data where the outcome is already known โ to train models to predict future outcomes. Common applications include:
- Predictive Modeling: Forecasting stock prices, interest rates, or credit defaults based on historical data. Algorithms like linear regression, support vector machines (SVMs), and decision trees are frequently employed.
- Credit Scoring: Assessing the creditworthiness of individuals or businesses by analyzing their financial history and other relevant factors.
2. Unsupervised Learning:
This involves analyzing unlabeled data to discover hidden patterns and structures. Useful applications in finance include:
- Customer Segmentation: Grouping customers based on their financial behavior to tailor marketing campaigns and product offerings. Clustering algorithms like k-means are commonly used.
- Anomaly Detection: Identifying unusual transactions or patterns that may indicate fraud. Techniques like principal component analysis (PCA) and autoencoders are valuable here.
3. Reinforcement Learning:
This approach trains agents to make optimal decisions in dynamic environments by learning from trial and error. It's gaining traction in algorithmic trading, enabling automated systems to learn and adapt to changing market conditions.
Practical Applications of Machine Learning in Finance
The theoretical concepts outlined above translate into numerous real-world applications across various financial sectors:
1. Algorithmic Trading:
High-frequency trading (HFT) firms extensively use ML algorithms to execute trades at optimal speeds and prices. Reinforcement learning is particularly promising in developing sophisticated trading strategies.
2. Risk Management:
ML models can analyze vast amounts of data to assess and manage various financial risks, including credit risk, market risk, and operational risk. This leads to more accurate risk assessments and better portfolio diversification.
3. Fraud Detection:
ML algorithms are exceptionally effective in identifying fraudulent activities, such as credit card fraud, identity theft, and money laundering. Anomaly detection techniques are crucial in this domain.
4. Portfolio Optimization:
ML can assist in building optimized investment portfolios that maximize returns while minimizing risk. Algorithms can analyze historical data and market trends to identify optimal asset allocations.
5. Regulatory Compliance:
ML is playing an increasingly important role in ensuring compliance with financial regulations. It can help automate compliance processes, detect regulatory breaches, and improve reporting efficiency.
Challenges and Considerations
Despite the significant potential, implementing ML in finance also presents challenges:
- Data Quality: ML models are only as good as the data they are trained on. Inaccurate, incomplete, or biased data can lead to flawed predictions.
- Model Interpretability: Understanding why a model makes a specific prediction is crucial, particularly in regulated environments. "Black box" models can be difficult to interpret.
- Computational Resources: Training complex ML models often requires substantial computational power and infrastructure.
Conclusion
Machine learning is revolutionizing the financial industry, offering unprecedented opportunities for enhanced efficiency, risk management, and profitability. While challenges remain, the ongoing advancements in ML algorithms and computing power are paving the way for even more sophisticated applications in the years to come. By understanding both the theoretical foundations and the practical applications, financial professionals can harness the power of ML to gain a competitive edge in this rapidly evolving landscape. Further research and exploration into specific algorithms and their implementations within various financial contexts will solidify a strong grasp of this transformative technology.
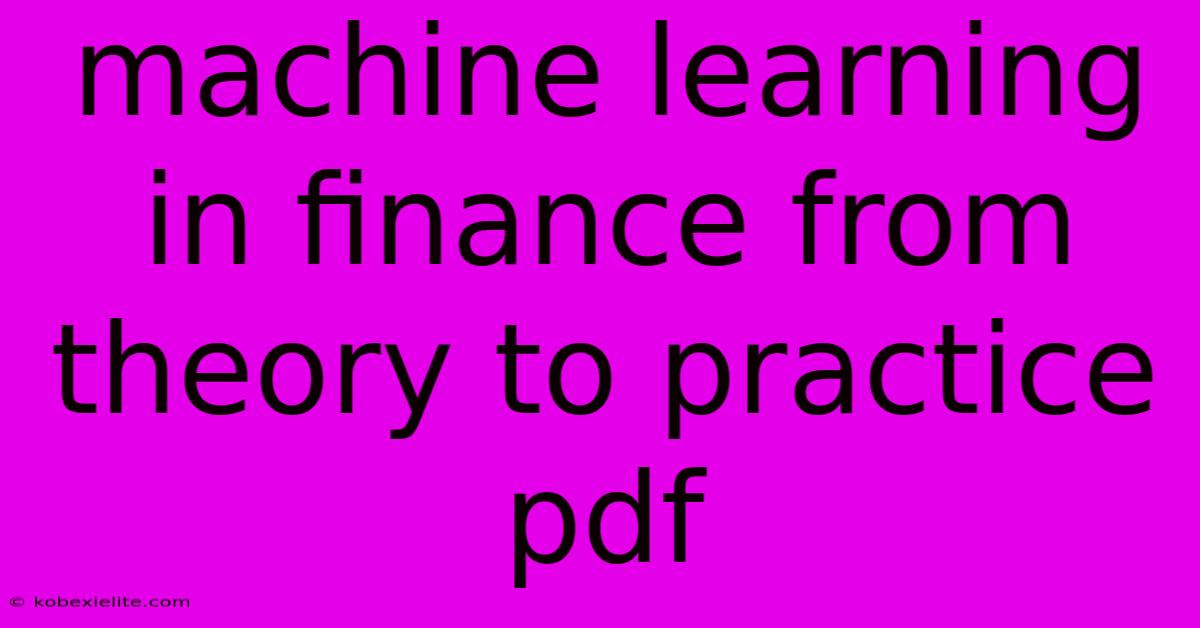
Thank you for visiting our website wich cover about Machine Learning In Finance From Theory To Practice Pdf. We hope the information provided has been useful to you. Feel free to contact us if you have any questions or need further assistance. See you next time and dont miss to bookmark.
Featured Posts
-
Incarnate Word Cardinals Vs Lady Of Lakes Live Stream
Dec 15, 2024
-
San Francisco Hit By Tornado Warning
Dec 15, 2024
-
Abbotsford 3 0 Victory Over Manitoba
Dec 15, 2024
-
Keyless Digi Ping New Integration
Dec 15, 2024
-
Stars Name Bbl 14 Squad
Dec 15, 2024