Ml For Finance
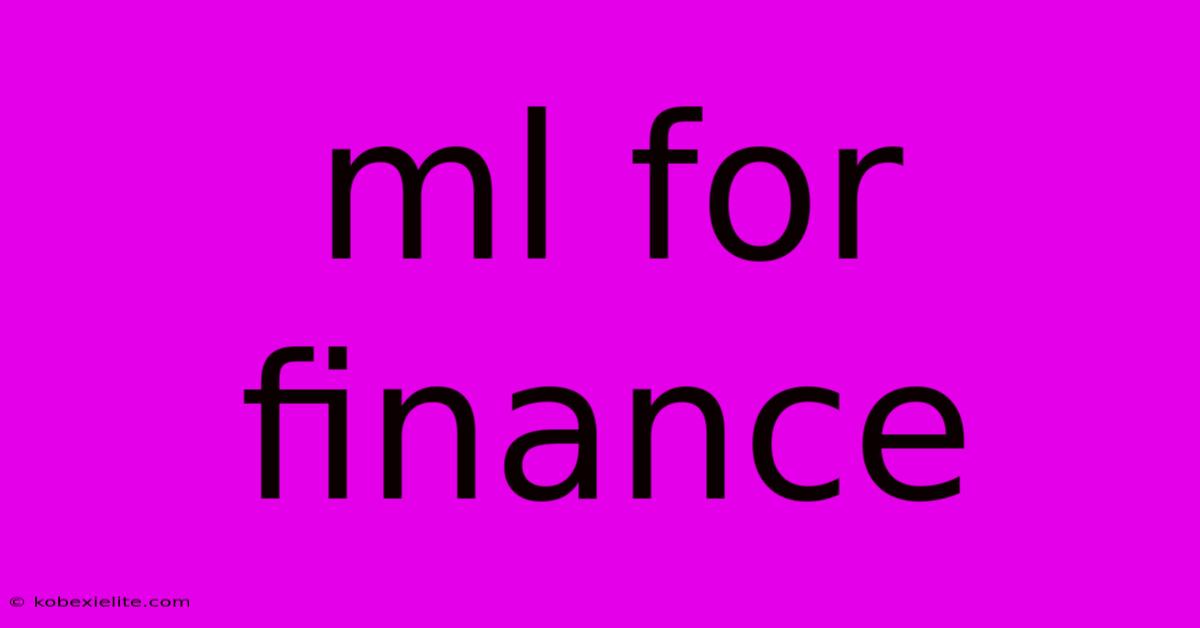
Discover more detailed and exciting information on our website. Click the link below to start your adventure: Visit Best Website mr.cleine.com. Don't miss out!
Table of Contents
ML for Finance: Revolutionizing the Financial Industry
The financial industry is undergoing a massive transformation, driven by the power of machine learning (ML). From algorithmic trading to fraud detection, ML is rapidly becoming an indispensable tool for financial institutions of all sizes. This article delves into the diverse applications of ML in finance, exploring its benefits and challenges.
What is Machine Learning in Finance?
Machine learning, a subset of artificial intelligence (AI), involves the development of algorithms that allow computer systems to learn from data without explicit programming. In finance, this translates to using historical and real-time data to build predictive models, automate processes, and gain valuable insights. This contrasts with traditional methods which rely heavily on pre-defined rules and human intuition.
Key Applications of ML in Finance:
1. Algorithmic Trading: ML algorithms can analyze vast datasets of market data (price movements, volume, news sentiment) to identify profitable trading opportunities in fractions of a second. This high-frequency trading (HFT) leverages speed and efficiency to maximize returns.
2. Fraud Detection: ML models excel at detecting fraudulent transactions by identifying patterns and anomalies in financial data. By analyzing historical fraud cases, these models can predict and prevent future fraudulent activities, saving financial institutions significant losses.
3. Risk Management: ML algorithms can assess and manage various types of financial risk, including credit risk, market risk, and operational risk. These models can predict potential defaults, optimize portfolio allocations, and improve risk mitigation strategies.
4. Customer Relationship Management (CRM): ML can personalize customer experiences by analyzing customer data to understand their needs and preferences. This enables targeted marketing campaigns, improved customer service, and increased customer loyalty.
5. Regulatory Compliance: ML can assist in meeting regulatory requirements by automating compliance processes, detecting potential violations, and generating reports efficiently.
Benefits of Using ML in Finance:
- Increased Efficiency: Automation of processes frees up human resources for more strategic tasks.
- Improved Accuracy: ML models can analyze data far more rapidly and accurately than humans, minimizing errors.
- Enhanced Decision-Making: Data-driven insights provide a stronger basis for informed decisions.
- Competitive Advantage: Early adoption of ML technologies can provide a significant competitive edge.
- New Revenue Streams: Developing and deploying ML-based financial products can generate new revenue sources.
Challenges of Implementing ML in Finance:
- Data Quality and Availability: ML models rely on high-quality, accurate, and readily available data. Lack of such data can limit the effectiveness of these models.
- Model Interpretability: Understanding why a model makes a particular prediction is crucial, especially in regulated industries. Many complex ML models (like deep learning) are "black boxes," making interpretation challenging.
- Computational Resources: Training and deploying sophisticated ML models require significant computational power and infrastructure.
- Regulatory Scrutiny: The use of ML in finance is subject to strict regulatory oversight, requiring careful compliance.
- Ethical Concerns: Bias in data can lead to biased models, raising ethical concerns regarding fairness and discrimination.
The Future of ML in Finance:
The future of ML in finance is bright. As data volumes increase and computational power improves, we can expect even more sophisticated applications of ML in areas such as:
- Personalized Financial Advice: AI-powered robo-advisors providing tailored financial advice based on individual circumstances.
- Predictive Analytics for Economic Forecasting: ML models predicting economic trends with greater accuracy.
- Decentralized Finance (DeFi): ML playing a critical role in the security and efficiency of decentralized financial systems.
In conclusion, machine learning is transforming the financial landscape. While challenges remain, the benefits of increased efficiency, improved accuracy, and enhanced decision-making are compelling. Financial institutions that embrace ML will be well-positioned for success in the increasingly competitive and data-driven world of finance. The continuous evolution of ML techniques promises even more groundbreaking applications in the years to come.
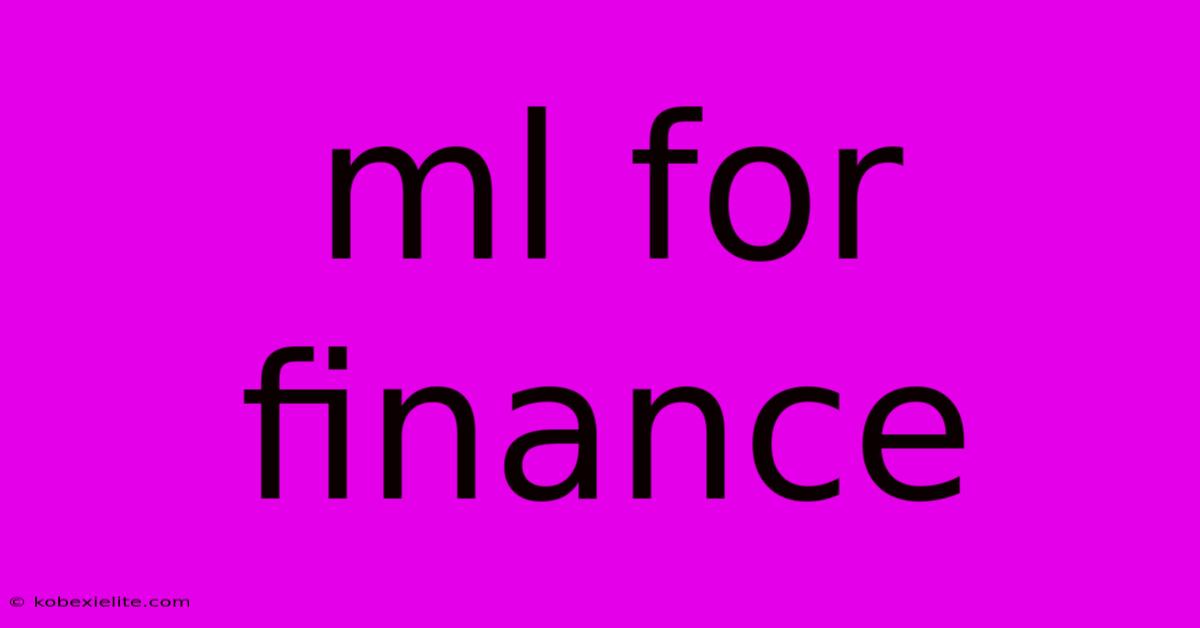
Thank you for visiting our website wich cover about Ml For Finance. We hope the information provided has been useful to you. Feel free to contact us if you have any questions or need further assistance. See you next time and dont miss to bookmark.
Featured Posts
-
Cfa In Finance
Dec 15, 2024
-
Finance Target Schools
Dec 15, 2024
-
Loyola Finance
Dec 15, 2024
-
Arsenals Title Dream Falters After Draw
Dec 15, 2024
-
City Finance Longview Texas
Dec 15, 2024